Keiran Paster
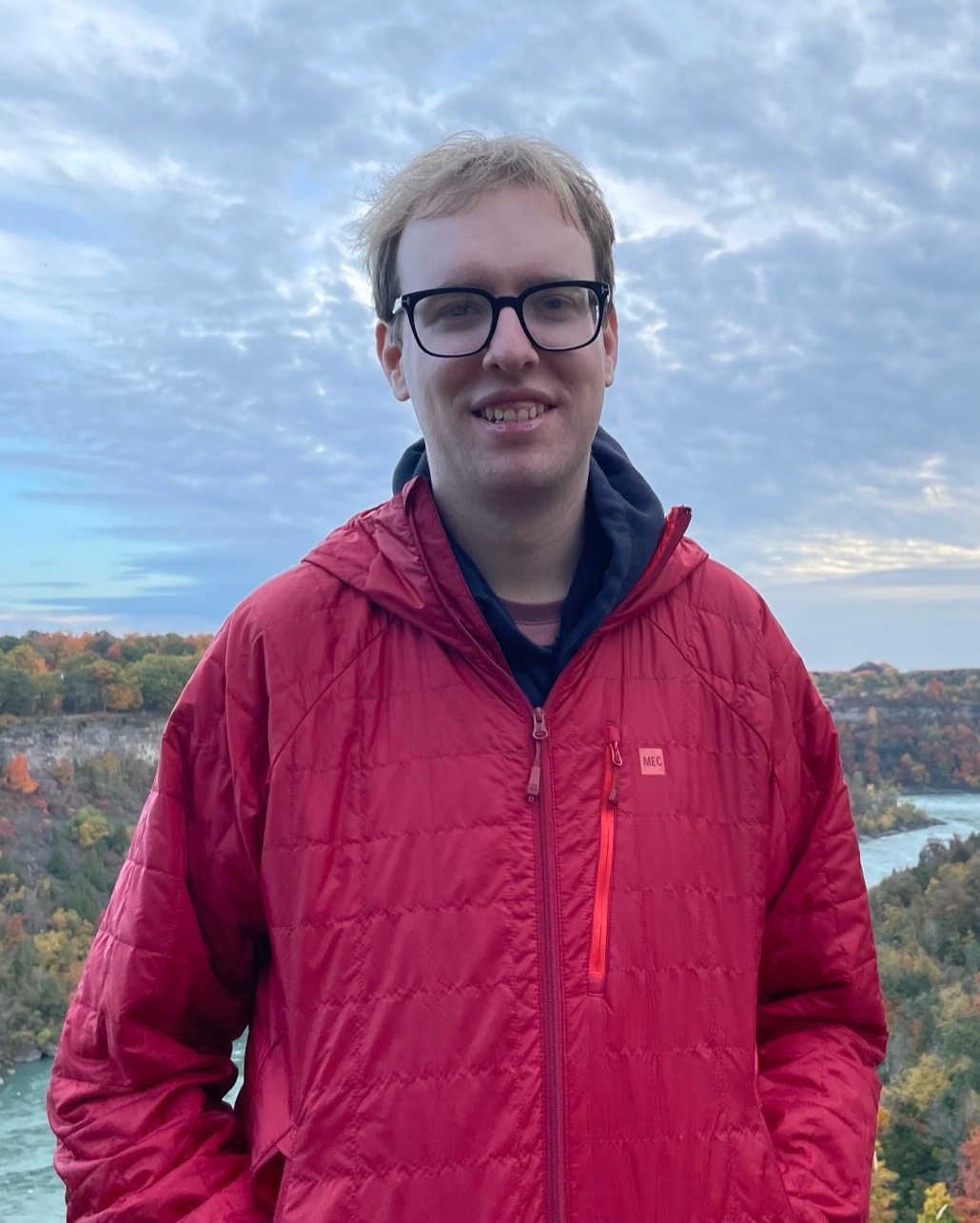
I am a fifth-year PhD candidate in the Department of Computer Science at the University of Toronto and I am currently on the job market.
- I am fortunate to be advised by Jimmy Ba and Sheila McIlraith.
- I am also affiliated with the Vector Institute.
- In Fall 2023, I was a student researcher at Google working on data efficiency for LLMs with the Gemini and Blueshift teams.
My research interests lie in AI reasoning and decision-making and my goal is to create AI systems that effectively scale with compute to process the world’s information and fast-forward scientific progress.
Research highlights from the last year include:
- Automatic prompt engineering, which shows for the first time that when given access to trial-and-error, LLMs can control themselves through prompting often better than a human can (resulting in the discovery of a prompting trick that gives insight into the psychology of GPT).
- STEVE-1, an instruction-following agent in Minecraft created using a novel methodology where we finetune a model pretrained on years of Minecraft videos on goal-relabeled data. STEVE-1 acts directly using keyboard and mouse input and follows open-ended text and visual instructions.
- OpenWebMath, 14.7B tokens of mathematical documents gathered from Common Crawl for use in LLM pretraining and midtraining. OpenWebMath improves mathematical reasoning performance over 20x more effectively per-token than general-domain data and has already been used to train several open and closed models.
- Llemma, the strongest open 7B and 34B base models for mathematical reasoning. These models are trained for up to 200B tokens primarily of OpenWebMath and show GPT-3.5-level performance with few-shot prompting even on held-out math evaluations.